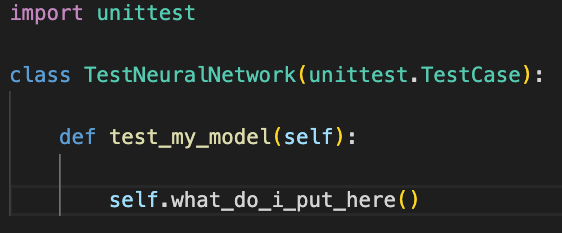
Si querés un tl;dr, la respuesta es sí.
Encuentro con frecuencia ingenieros que piensen que no hay razón de escribir unit tests para código de aprendizaje automático o que no pueden imaginar en donde se necesita un unit test. Generalmente, la idea que tienen es "Solo hay un modelo. Se da datos al modelo y se recibe una respuesta. No hay nada que probar." He trabajado en aprendizaje automático para unos años ahora y frecuentemente encuentro situaciones en que un unit test puede ahorrar un montón de tiempo. Dejáme explicar unas razones de usar unit tests un tu código.
Unit Tests Ayudan a Hacer el Código Reusable
Esto sería más o menos claro par a la mayoridad de ingenieros, pero si el código es reusable, se puede hacer más proyectos más rápidamente. Unit tests te ayudan pensar en problemas que puedan ocurrir y hacer que tu código funciona en todos los circunstancias que supones. Esto puede prevenir problemas como tu proyecto funcionando perfectamente por un dataset, pero no el otro.
Unit Tests Encuentran a Cambios que afectan a la funcionalidad
Si trabajas con un grupo de gente o si volvés a un proyecto que estabas haciendo antes, puede ser que hay situaciones en que no recuerdas o conoces el código muy bien. En estas situaciones, es posible que hacés (o un miembro de tu grupo hace) un cambio que inadvertidamente rompe a la funcionalidad del código. En unos caso, esto puede pasar de una manera no tan claro. Si hacés unit tests para la funcionalidad, cuando hacés un cambio, podés averiguar que todo funciona bien por correr los unit tests.
Unit Tests Ahorran Tiempo
Have you ever written a training script with a dataloader and a model and run it on your data only to have an error happen that you then had to trace back and fix? Or maybe it was even worse, you launched an Azure cloud job with your script running inside it, only to come back 10 minutes later and see it failed. If you have then repeated this step multiple times as you keep going back and debugging, then hopefully you have already seen my point. Unit tests allow you to test the functionality of your ML components quickly without needing to put all the components together and load actual data.
When to Write Unit Tests
So now that we have established that it is beneficial to write unit tests, you might be wondering where exactly then can they be applied for ML code? This is not an exhaustive list, but here are some of my recommendations:
Anywhere there is an exception
When building a Pytorch dataset, you may want to add some exceptions for things like when an annotation file doesn’t exist or when there are no images in a folder. It is important that we know these exceptions work so that when someone uses the dataset, the exception properly tells them what they did wrong. Likewise, you may have an exception in a model that tells you when the configurations are not set properly. If you wait until you run the code to test whether it works or not, it may take a long time before you actually see the exception work (or figure out it isn’t working).
For inputs and outputs
Machine learning is all about inputs and outputs. If your data loader is outputting the wrong format, you’ll never get it to work with your model and if your visualization function doesn’t accept the shape of the output from your model, you won’t be visualizing anything. Take a minute to write unit tests that check the input and output of your various ML components.
When you have custom logic
If you ever write your own components, like a loss function, a special layer or maybe a post processing step, it is always a good idea to make some tests to check if it is working correctly. This doesn’t need to be exhaustive, because it can be next to impossible to write tests that cover every possibility. However, you can certainly cover a couple of cases to have some peace of mind that things are working the way you expect them to.
From one ML engineer to another, I highly encourage you to learn more about unit tests and look for other areas of your code that might benefit from it. There are tons of great resources out there on how to use, write, and debug using unit tests and this information is not exclusive to standard software engineering. Next time you write an dataset or a neural network, try some of the tips I listed above. If you benefited from it (or not), please let me know in the comments!
For more articles on Python, check out some of my other content.
Deja un comentario
Lo siento, tenés que estar conectado para publicar un comentario.
Connect with
Login with Linkedin